As data has come to dominate our world, perhaps no industry has been more transformed than investing. Where analysis was once an inexact art, cold hard numbers now rule the day. Pioneering “quant jocks” were early to realize data held the key to cracking the market’s code. Armed with computing power and voracious appetites for information, they developed algorithms that could outthink Wall Street’s elite.
What started as a covert endeavor in darkened rooms has exploded into the mainstream. Machine learning now guides trillions in assets, with quants populating banks, hedge funds, and beyond. Yet one arena remains a true frontier – the Wild West of cryptocurrency. The blockchain unleashes unprecedented transparency, a treasure trove even more vast than traditional markets. It’s here the boldest quant visionaries are staking their claims, designing digital strategies to profit from Bitcoin’s every byte.
This story by
Finestel explores how data dominoes fell to bring us to this revolution’s cutting edge. From seminal strategies to players pushing limits, its aim is to decode the quant quest – and shine a light on cryptos’ quantitative future. So dive in to learn how algorithms now answer the age-old question: What moves markets next? The answer, like money itself, has gone digital – and it’s guided by ones and zeros alone.
What is Quant Trading? Turning Data into Dollars
Quantitative trading transforms investing from an art to a science. It employs mathematical and statistical modeling to analyze market data methodically and make calculated trading decisions. Quant traders develop algorithms that run on high-octane computers to identify opportunities and execute trades with machine precision.
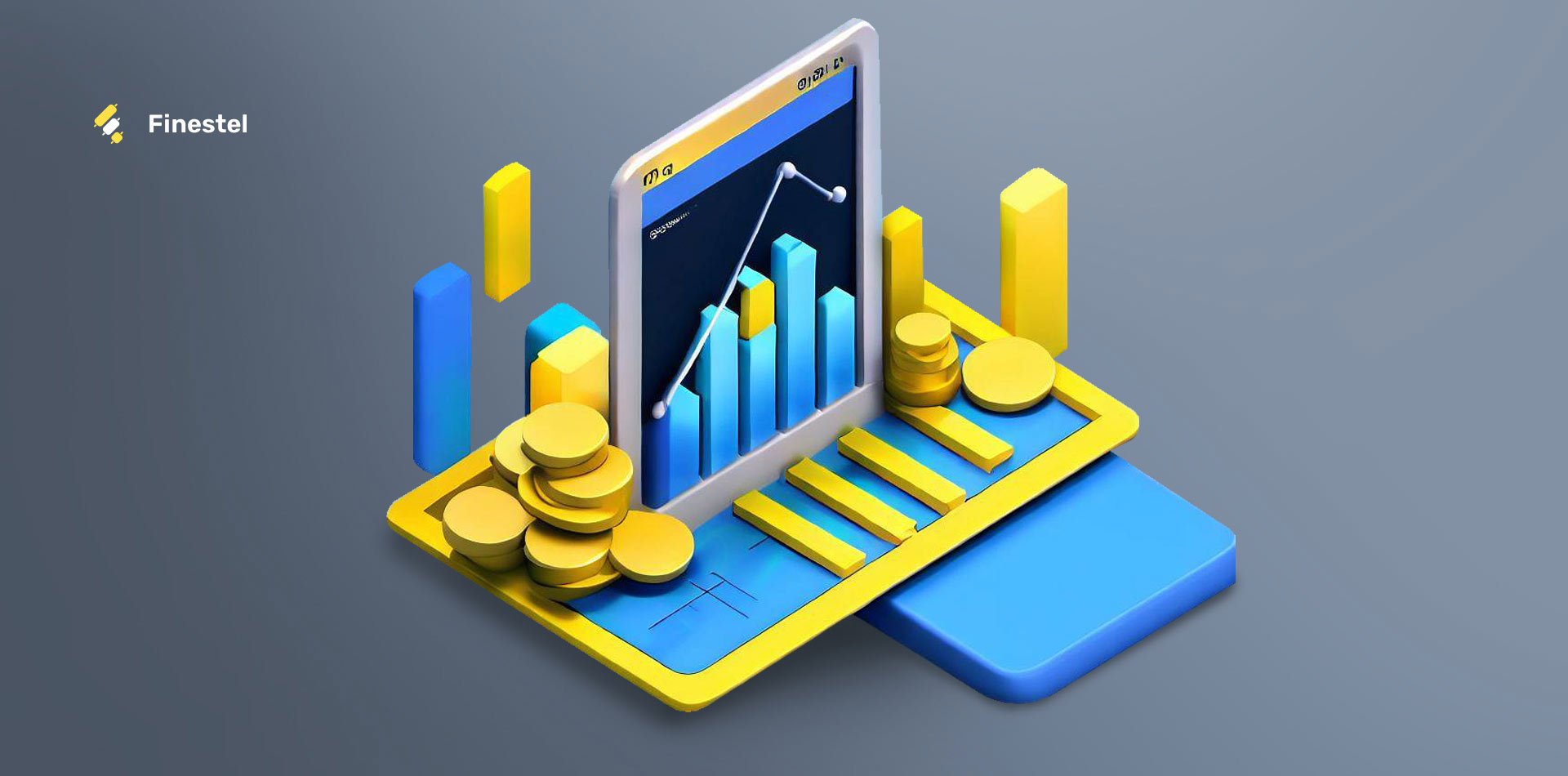
Gone are the days of relying on gut feelings or emotions. Quant trading injects cold hard logic into the investing process. These strategies analyze everything from price movements to volatility to social sentiment. It’s about letting the data do the talking.
Strategies range from short-term statistical arbitrage to longer-term factor-based investing. But the hallmarks are backtesting hypothetical models against historical data to prove efficacy, automating the system for emotionless execution, and optimizing relentlessly as new data emerges.
It’s a methodical Moneyball approach, using technology to gain an edge previously reserved for Wall Street power players. In quant trading, the numbers don’t lie. If your algorithms have the sharpest predictions, you reap the rewards. It levels the playing field for quants who master data.
The Evolution of Quant Trading
Quant trading has exploded over the past decades thanks to computers and data. In the 1970s, trading was mainly fundamental or technical analysis done by humans. However, pioneers realized math and statistics could give them an advantage.
They began developing models to identify market inefficiencies. It was like card counting at casinos – using science to shift the odds in your favor. Early quants even hid their computers behind closed doors, knowing they had stumbled upon a goldmine.
As technology advanced, so did quant trading. Soon automated execution and high-frequency systems emerged, multiplying returns through sheer speed and efficiency. No humans could match it.
Next machine learning leads to adaptive systems that continuously improve predictions. Quant trading had evolved from a niche strategy to dominating markets. Today over 70% of trading across assets utilizes algorithms.
And the machines keep getting smarter. With alternative data, AI trading, and quantum computing on the horizon, quant trading shows no signs of slowing. The players may change, but the game stays the same – using data to gain an edge in the arena. Quants embrace progress to perfect their craft.
Quantitative Trading Vs. Algorithmic Trading
In summary, algorithmic trading provides the pipelines while quants provide the fuel. Algo traders systemize the process while quants supply the trading intelligence. The symbiotic integration of these two disciplines is key to success in modern electronic trading.
|
Algorithmic Trading |
Quantitative Trading |
Focus |
Automating execution of orders based on conditions |
Developing predictive trading signals and models |
Goal |
Efficient entry and exit of trades |
Alpha generation and strategy optimization |
Approach |
Programmatic automation |
Statistical modeling and analysis |
Key Activities |
Systematizing order types, routing, timing |
Researching new signals, backtesting, portfolio optimization |
Competitive Advantage |
Speed, cost efficiency |
Informational edge from models |
Workforce |
Software developers, programmers |
Data scientists, quant researchers |
Risk Management |
Guardrails, automation limits |
Diversification, position sizing |
Time Horizon |
Any, but often shorter term |
Any, but often longer term |
Quant Trading in the Crypto Market
Quantitative trading in cryptocurrency has unique aspects that set it apart from other financial markets. While the basic strategies are similar across assets, the quant
crypto trading strategy adds new dimensions.
One is the rich data from the blockchains themselves. By analyzing transaction patterns, we can glean insights into how major players like exchanges and whales manipulate the market. This offers signals traditional quant models lack.
Crypto also has programmable assets through decentralized finance. Not only can quant strategies make predictions, but they can now interact directly with financial primitives like lending through smart contracts. This automation allows strategies traditional funds can only observe.
Risk is another differentiator. Besides price volatility, crypto faces risks from technical events like forks or hacks. Its digital nature introduces new threats outside standard risk models. As the space evolves, quant models must adapt to these unique risk factors.
In many ways, cryptocurrency arrived at the perfect time. It debuted as quantitative analysis rose to prominence. While still early, crypto lends itself well to cutting-edge automated trading through its blockchain data and programmable assets. This convergence of technology and market conditions makes it fertile ground for innovative quant strategies unlike anything seen before.
How Quant Strategies Work: Teaching the Machines
Quantitative trading employs a variety of sophisticated approaches. But they all rely on data-driven backtesting to prove efficacy. Let’s explore some prominent quant methodologies:
Statistical Arbitrage
This strategy analyzes the historical price movements between similar assets to identify short-term divergences. When a strong correlation suddenly weakens, arbitrageurs pounce to profit from the expected convergence back to normal levels. By simultaneously buying one security while shorting another, gains are made as the pricing anomaly resolves.
The prices of Bitcoin and Ethereum often move in tandem due to their correlation as the two largest cryptocurrencies. However, in August 2022 Ethereum rose 20% while Bitcoin fell 5% for no clear reason. A quant model detected this divergence from their normal 0.8 correlation and shorted Bitcoin while going long Ethereum, profiting from the expected convergence back to their relationship.
Momentum
Momentum traders study indicators like 50-day moving averages to spot assets with powerful short-term price trends. Machine learning algorithms crunch numbers from the past to predict which stocks remain bullish, representing future buying opportunities. By following the herd, this strategy capitalizes on the tendency of trends to persist in the near term.
After Tesla announced it would accept Bitcoin payments in January 2021, the price of BTC surged over 300% within a month. A momentum strategy identified this parabolic move and opened a long position. Riding the powerful trend, the strategy doubled its money as Bitcoin peaked at over $60k that year.
Mean Reversion
Taking a contrarian view, mean reversion investors analyze price data to identify when an asset’s value has wandered too far from its long-term average. The assumption is that over time, prices will “revert to the mean”. Statistical models pinpoint overbought and oversold anomalies to profit as equilibriums are restored.
During the ‘crypto winter’ bear market of 2018, many small altcoins lost 90% of their value. One such coin, Litecoin, had plunged to just $20 from an all-time high of $400 the prior year. A mean reversion quant saw an opportunity for a bounce and established a long position. Sure enough, Litecoin rebounded 60% over the following 6 months as the strategy profited.
High Frequency Trading (HFT)
Using algorithms, fiber optic networks, and co-located servers within milliseconds of exchange matching engines, HFT players transact enormous order volumes lightning-fast. By scalping tiny bid-ask spreads many times per day, they exploit momentary price distortions across assets. Speed is their sole advantage in this cut-throat game.
Multi-Factor
Rather than relying on one factor like value or momentum, multi-factor strategies combine the predictive power of multiple indicators through advanced optimization. Machine learning determines each factor’s optimal weighting depending on the market environment, balancing upside with stability.
The common thread uniting all quant strategies is letting reams of historical data point the way to trades with a positive expected value. Through rigorous backtesting, the machines learn to recognize profitable patterns and crack the market’s code. Automation then takes over, executing with consistent discipline – and the numbers don’t lie.
Who Is Quant Trading for?
Quantitative trading was once the realm of secretive hedge funds and banks. But today a growing universe of investors and institutions utilize these data-driven strategies. Let’s examine who’s joining the quant revolution:
- Hedge Funds – Renaissance, Two Sigma, D.E. Shaw. The top quant funds boast some of the most profitable long-term track records through their advanced modeling and technology. Their data sets and brainpower are immense competitive advantages.
- Investment Banks – Banks apply quant techniques for activities like algorithmic trading, liquidity provisioning, and exotic derivatives pricing. Physics PhDs team with traders.
- Asset Managers – Employ quants for portfolio optimization, risk management and high frequency trading. Some offer quantitative fund products directly to investors.
- Prop Trading Firms – Proprietary shops like Jump Trading and Tower Research trade their own capital through advanced statistical arbitrage and HFT strategies. Trading is their only business.
- Individual Traders – Online platforms like QuantConnect allow retail investors to access institutional-grade quant tools for backtesting and automation. Data availability democratizes quant trading.
Across all types of institutions, expect quant finance to keep expanding. As data proliferates, those who can harness it have an edge. Quants are the interpreters between data and dollars. Their machine whispering converts bits into bounty. The rise of the machines brings riches to their masters.
Getting Started with Quant Trading as an Individual
For individuals interested in breaking into quant trading, the journey begins by gaining proficiency in programming and quantitative skills. Focus on learning one of the main languages used in finance like Python or C++, as being able to code is fundamental.
Spend time studying topics in statistics, mathematics, financial economics, and machine learning to develop a conceptual understanding of quantitative modeling techniques.
Once you have built a strong foundation in programming and quantitative theory, start experimenting with backtesting trading strategies on paper trading platforms. A great free resource for this is Quantopian, which allows you to develop and test strategies using historical market data, without any real money at risk. As strategies are developed, refine them through rigorous backtesting and optimization to prove their efficacy.
In parallel, build your own simulated trading environment or “lab” at home to practice automating the execution of strategies and monitoring their performance over time. This will help prepare you for the technical aspects of live trading. Only after extensive paper trading should you consider funding a small live account to begin trading with real capital. Start modestly and prove your approach works in live markets before scaling up position sizes.
Throughout the learning process, focus on continual improvement by reviewing strategies after significant market events and remaining up to date on new techniques. With a dedication to developing your skills through practice, experimentation, and refinement over time, individuals can certainly break into quant trading, but it requires a long-term, scientific mindset and commitment to the craft.
Assembling a Quant Team
It takes a diverse ensemble of talent to design, build, and operate a world-class quant trading strategy. Let’s explore the key players:
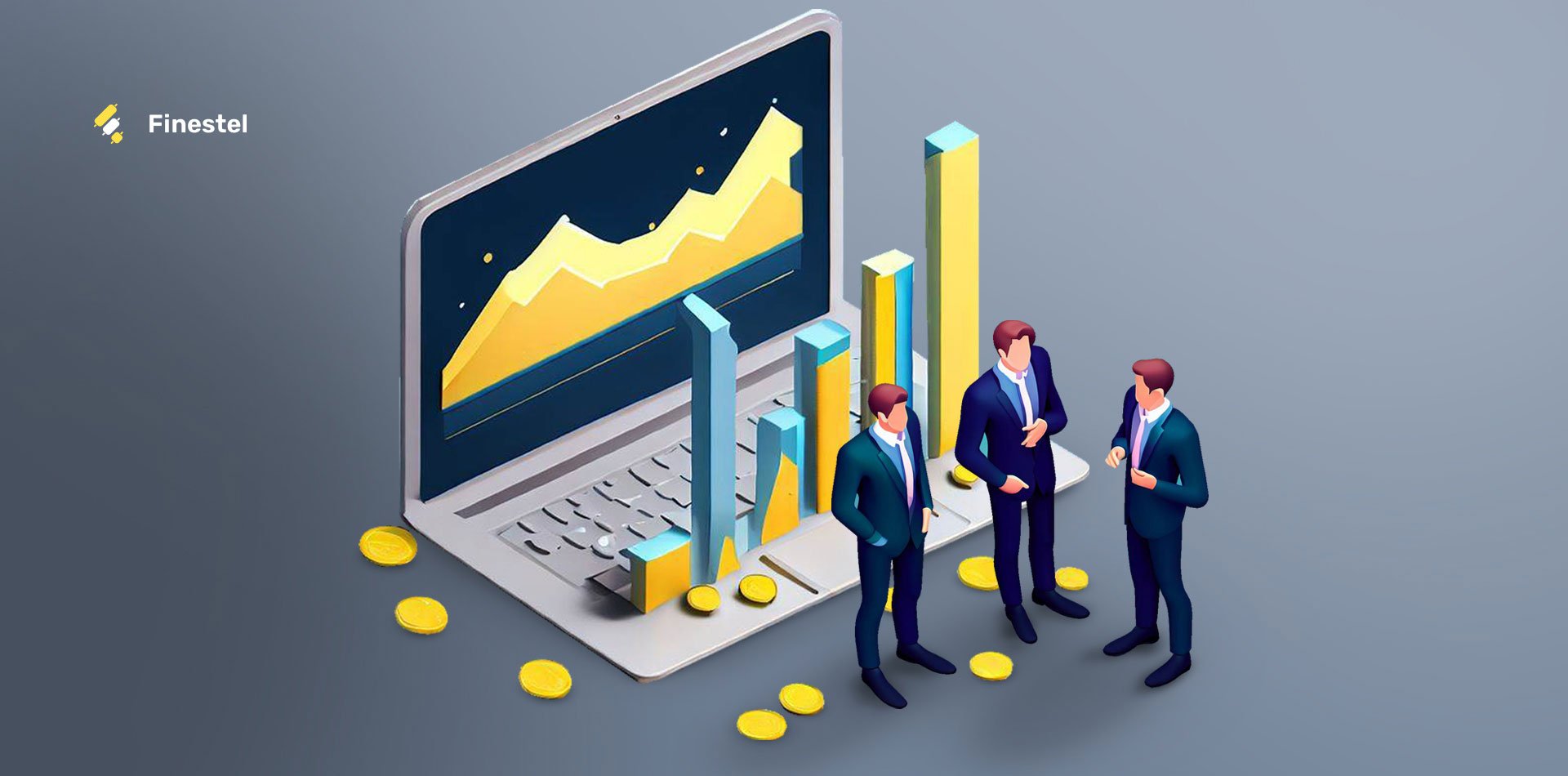
- Quantitative Researchers – These quants are architects, creating the predictive models and algorithms that drive the strategy. Combining math, statistics, and finance, researchers backtest hypothetical models before passing them to engineers.
- Software Developers – The tech wizards who build the pipelines to feed data into models and turn signals into trade execution. Fluent in languages like Python and C++, they make sure systems run seamlessly.
- Portfolio Managers – The conductors overseeing the trading orchestra. Portfolio managers interpret model outputs and fine-tune strategies to maximize returns while minimizing risk.
- Risk Management – The guard rails that keep operations safe. Risk analysts monitor positions and market conditions to ensure appropriate controls and limits are in place.
- Data Scientists – Providing the fuel that powers quant trading – data. Data scientists organize, clean, and preprocess data to train algorithms. Their machine-learning expertise is invaluable.
Though their skills differ, together the Quant Avengers assemble a formidable market force. When the team works in harmony, the profits come flowing in.
Capital Requirements for Starting Quant Trading
The amount of starting capital needed to implement different quantitative trading strategies successfully can vary significantly. As a general guideline, most statistical arbitrage or market-neutral strategies that rely on holding offsetting long and short positions require a minimum of $100,000. This allows for adequate diversification and capitalization of positions.
For highly liquid intraday trading strategies, it is preferable to have at least $500,000 in order to deploy positions with sufficient size across multiple stocks, futures, or forex pairs. With less capital, frictional costs like commissions may erode profits substantially.
The highest capital requirements are needed for high-frequency trading (HFT) strategies that rely on ultra-low latency technology and rapid order execution. Most HFT firms demand a minimum of $1-5 million to capitalize the infrastructure, operations, and trading systems required. Less capital makes it difficult to generate meaningful returns from these fast-paced approaches.
In all cases, starting with excessive leverage is ill-advised, as quant strategies are best served with sufficient capital to withstand short-term market fluctuations and volatility without jeopardizing the overall portfolio.
Key Challenges Building a Quant Business
Constructing an institutional-grade quant trading business requires immense investment and planning across many fronts:
Data Infrastructure and Tooling
Robust data pipelines must ingest, process, store and serve the terabytes of historical and streaming data fuelling strategies. Clean, accurate data is quant fuel. Cloud platforms help manage this data at scale. Specialized analytics tools like MATLAB and R provide visualization and modeling capabilities.
Specialized Talent Acquisition
Quantitative researchers, data scientists, quants developers – this specialized talent doesn’t come easy. Competition from top firms leads to astronomical compensation packages. Attracting and retaining talent means emphasizing state-of-the-art technologies, collaborative team environments, and appealing workplace culture alongside competitive pay.
Technology Management
Low-latency networks, co-located servers, adaptive infrastructure, and integrated software stacks – these are the high-performance engines executing quant strategies seamlessly. Systems must be continuously monitored, upgraded, and optimized by IT specialists to prevent performance degradation or costly outages.
Regulatory Oversight
As algorithmic trading expands, regulators intensify scrutiny of practices like high frequency trading. Robust controls, testing procedures, and compliance regimes are crucial to avoid fines or trading suspensions due to improper activities. Legal and compliance teams ensure quant businesses operate ethically and legally.
The barriers to launching a successful quant operation are sky-high. But the potential rewards make it all worthwhile. With meticulous planning and flawless execution, your algorithms can strike it richly.
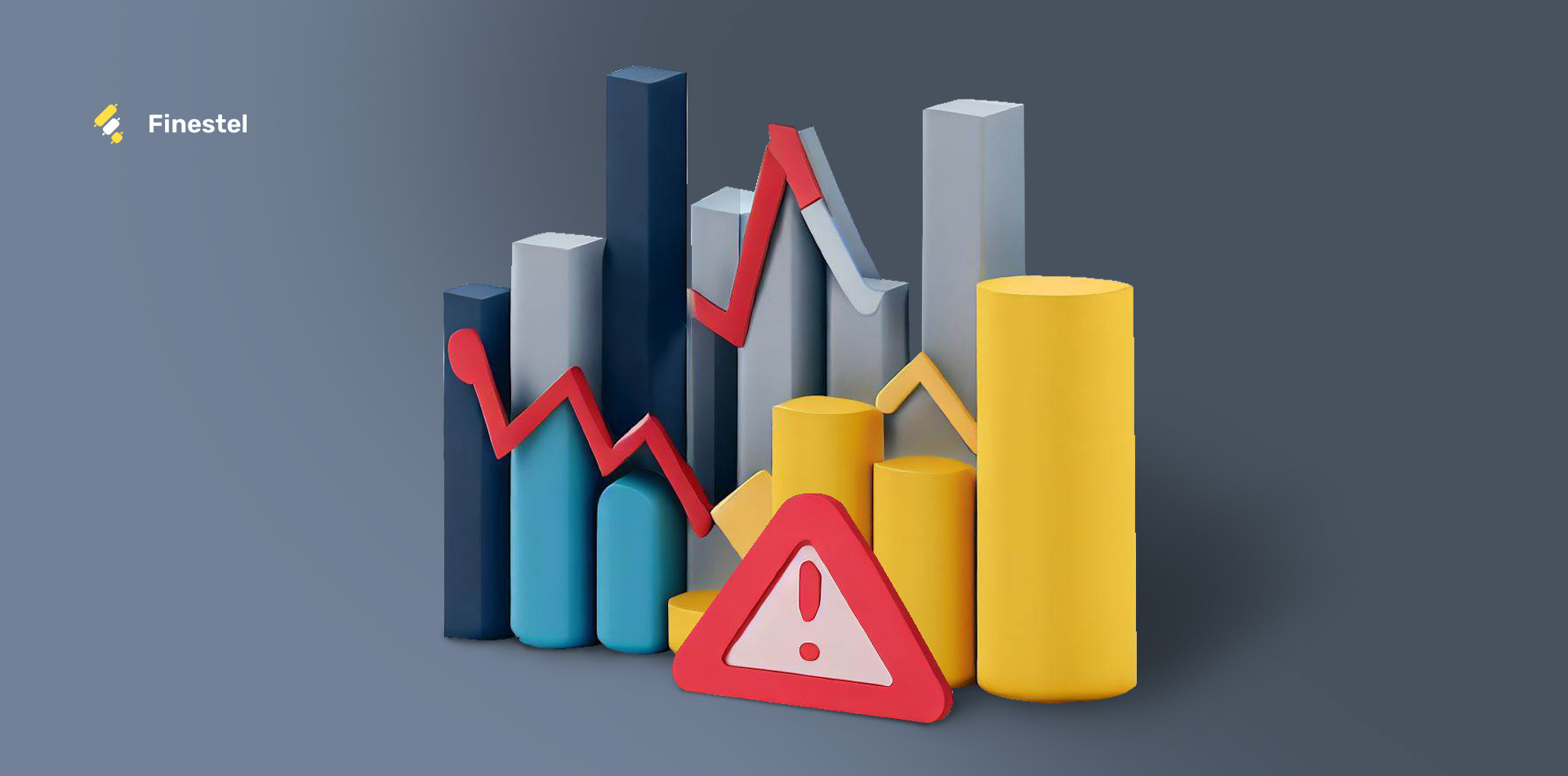
Quant Trading Tools
Quant traders leverage a variety of specialized software platforms and programming languages to develop, analyze, and execute their data-driven strategies. Popular backtesting environments like Quantopian, QuantConnect and TradeStation allow strategies to be coded and tested using historical market data, without any real money at risk. This simulation enables rigorous optimization and refinement.
Traders also rely heavily on statistical programming languages like MATLAB, R and Python to analyze reams of financial time series data and build predictive quantitative models. These flexible languages contain powerful libraries for tasks like data wrangling, machine learning, and algorithmic trading.
For live, automated execution, many quants integrate broker APIs into their workflows. Firms like Alpaca, Interactive Brokers, and Tradier offer low-cost programmatic trading interfaces. Strategies can be deployed to buy and sell in fundamental markets based on model signals.
Major cloud platforms, from Amazon Web Services and Microsoft Azure are also commonly used to manage the vast computing infrastructure required. Their elastic cloud environments seamlessly scale to handle the storage and processing of massive datasets powering modern quantitative strategies.
Top 5 Quant Trading Platforms
Quant trading platforms allow quantitative investment firms and traders to develop, test, and deploy algorithmic trading strategies. These platforms provide tools for backtesting trade ideas on historical market data, paper trading to simulate real trades, and live trading of strategies.
They also have features for monitoring portfolio performance, risks, and strategy profitability in real-time. Quant platforms typically offer APIs to pull market data feeds and transmit orders directly to brokerages. They provide a complete solution for quantitative finance professionals to transform their strategies into live, automated trading systems.
-
Citadel is a true powerhouse in the quant trading world. Since its founding in 1990, it has produced average annual returns of 19% through a blend of cutting-edge technology and sophisticated algorithms. Their high-frequency trading systems can blast through thousands of orders per second, giving Citadel an edge in today’s lightning-fast markets.
Beyond speed, Citadel also distinguishes itself with relentless innovation. Their state-of-the-art infrastructure seamlessly processes vast troves of market data to inform strategies and decisions. It’s no wonder they’ve dominated quant trading for over 30 years.
-
Founded in 1975, Bridgewater has quietly built one of the strongest long-term track records in the industry. Under visionary Ray Dalio’s leadership, their flagship Pure Alpha fund has returned around 12% annually since inception.
Operating with over $150 billion in assets, Bridgewater bases its success on innovative concepts like risk parity and balanced portfolios. Their culture of transparency and meritocracy also fosters the best ideas. Bridgewater proves that steady, risk-aware investing can thrive even in volatile times.
-
Co-founded in 1998, AQR helped pioneer the “factor investing” approach we see widely used today. By targeting specific market drivers like value and quality, their strategies have yielded significant returns for clients over the years.
AQR also distinguishes itself through academic-style research and transparency. They freely share insights to cultivate trust while identifying profitable factors through sophisticated algorithms and analysis. It’s no surprise they’ve grown to over $140 billion in assets.
-
Founded in 1988, D.E. Shaw blazed a trail by blending cutting-edge math, technology, and financial expertise. They sift through immense data troves to uncover opportunities using proprietary algorithms.
D.E. Shaw also led the way with the early adoption of high-frequency strategies. By leveraging advanced technology and lightning-fast execution, they achieved unprecedented efficiency and precision. It’s no wonder they’ve delivered average annual returns of 13.9% throughout their history.
-
Renaissance takes quant to another level. Founded in 1982 by renowned mathematician Jim Simons, they’ve achieved average annual returns of 39.1% through intensely mathematical models.
Renaissance employs hundreds of Ph.D.s and scientists to continuously refine complex predictive models. This interdisciplinary approach explores markets from diverse angles for unique insights. While famously secretive, the Renaissance’s success speaks for itself.
Conclusion
As data floods financial systems in ever-growing deluges, quants will stay swift on its tides. Their mission remains constant – surfing information waves to gain an edge. Yet the medium is sure to evolve. Tomorrow’s titans may emerge from fields like quantum computing, artificial general intelligence, and even sci-fi frontiers like brain-machine interfaces.
Wherever technology leads, cryptocurrency will likely remain the wild frontier. Its transparency offers unfiltered insights and a live laboratory to test trading ideas without bounds. There, the boldest visionaries are sure to push limits and dream up digital strategies never seen before. While building robust quant powerhouses presents immense challenges, the potential rewards make the effort worthwhile.
As algorithms continue refining their craft, one thing is clear. Data will remain the universal language of markets, a lingua franca understood by man and machine alike. In this new age, it falls to quants as interpreters to transform terabytes into treasure through trading innovation. Their mission moves ever forward – decoding financial systems one byte at a time.
Leave a Reply